Artificial-intelligence models are getting smarter, but they are also getting hungrier. Training a frontier-size model now requires millions of GPU hours; running it at scale keeps those accelerators lit 24/7. Data-center electricity use, already about 1.5% of global demand, is only beginning its climb. The International Energy Agency projects it to more than double to roughly 945 TWh by 2030—about as much power as Japan uses today—with AI the single biggest driver. The world’s grids might begrudgingly support that load, but the physics is blunt: most of the watt-hours go not to number-crunching, but to shuttling data between memories and processors.
This traffic jam—the von Neumann bottleneck—means we burn energy moving bytes back and forth, then burn almost as much again to cool the heat we just made. By contrast, the human brain performs the same class of pattern-recognition tasks while sipping about 20 W, roughly the power of a refrigerator light bulb. The brain’s trick is architectural: neurons store and compute in one place and only fire when their inputs change. No wasted cycles, no long-haul data buses.
Neuromorphic computing copies that playbook. In hardware, it packs millions of tiny “neurons” beside equally tiny, tunable “synapses,” often built from non-volatile memories such as ReRAM or MRAM. In software, it trades today’s frame-by-frame matrix math for spiking neural networks, where information travels as time-stamped pulses. The result is event-driven silicon that stays idle—and cool—until the outside world gives it something worth noticing. Because compute and memory share the same fabric, every spike updates weights locally, slashing the energy otherwise lost in traffic.
The technology is still speculative, but the prize is immense. If spiking-based chips can deliver even a tenth of the brain’s staggering 20 peta-flops-per-watt efficiency, they would unlock always-on AI without drowning the planet in heat or energy bills. In this report, we highlight the top neuromorphic computing stocks to watch, curated for pure-play exposure to this brain-inspired solution to AI’s growing power bottleneck.
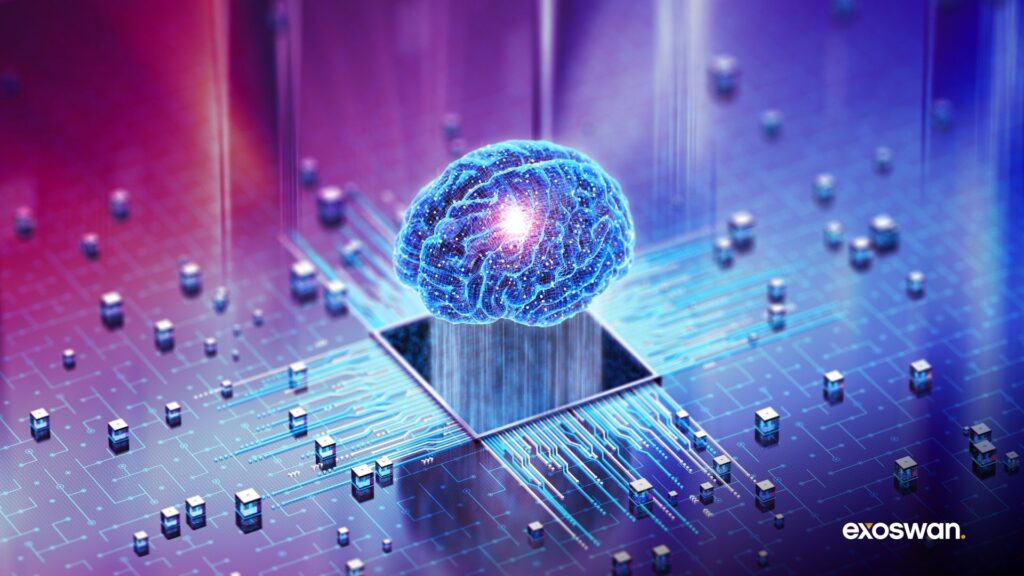
How do neuromorphic chips differ from familiar “AI chips”?
GPUs, TPUs, and other deep-learning accelerators are indeed inspired by the brain, but only at the level of math: they multiply dense matrices of floating-point numbers to imitate neuron activations. Under the hood they still run in lock-step, move bytes between distant SRAM and DRAM, and waste energy computing zeros. Neuromorphic hardware goes a step closer to biology. Spiking neurons send information only when something changes, so most of the circuit is idle most of the time. The result: prototypes such as Intel’s Loihi 2 or IBM’s NorthPole show 10- to 100-fold energy cuts on tasks like keyword spotting or anomaly detection.
That said, the two worlds are not mutually exclusive. Classic AI accelerators would still dominate tasks where training data is abundant and cloud power is cheap—large language models, photorealistic image generation, billion-node graphs. Neuromorphic hardware would slide into niches where power, latency, or adaptability are the bottlenecks. In future devices, the two will likely coexist: a cloud-trained model distilled down to a spiking network for local, real-time execution; or a neuromorphic pre-processor that filters raw sensor streams before handing higher-level reasoning to a GPU.
Pure-Play Neuromorphic Computing Stocks
Pure-play neuromorphic computing stocks sit at the bleeding edge of hardware innovation. These tiny companies sell nothing but brain-inspired chips, so their share prices swing on every lab milestone and customer pilot. They give investors the cleanest exposure to spiking-network silicon—without the dilution of broader semiconductor portfolios—but they also carry binary risk: each design win or funding round can double or halve the valuation. Only one public company is currently in this tier, underscoring just how early we are in the neuromorphic computing market.
BrainChip Holdings (ASX: BRN)
HQ: Australia; Pure-play in digital spiking neural processors for edge AI.
BrainChip is a small Australian chip company with one big idea: put a brain-like computer at the sensor itself. Its Akida processor is a fully digital, event-driven neural network that only “fires” when an input changes, so tasks such as keyword spotting, vibration analysis or security vision can run for months on a coin-cell battery. Earlier this year, the firm released a second-generation core that adds vision-transformer layers and temporal event networks and began shipping the design on a simple M.2 card so engineers can test it in any PC.
The bullish view is direct. Akida already ships in plug-and-play modules and a growing set of microcontroller boards; each volume design win could turn into a royalty stream backed by the MetaTF software tool-chain. Because power efficiency is measured in microwatts the chip competes where GPUs never fit—hearing aids, industrial sensors, drones. Patents and first-mover status give BrainChip room to run if neuromorphic demand finally arrives.
Yet the risks are plain. Annual revenue is still tiny and R&D burn exceeds sales, so dilution is the default funding source. Bigger semiconductor houses can cross-subsidize their own spiking-network blocks and bundle them for free. The technology also forces customers to rethink algorithms; without a larger field team, design cycles can drag for years. Buying the stock therefore means betting that 2025–2027 will bring visible product launches, not just more press releases. If that catalyst materializes, the company’s tiny market cap could re-rate sharply upward for investors.
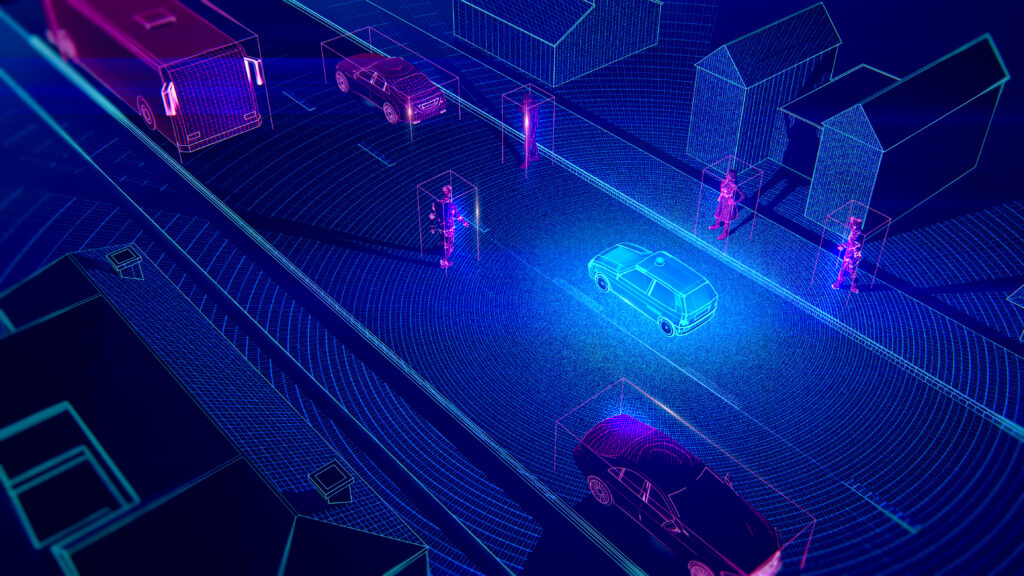
Memory & Compute-in-Memory Enablers
Memory and compute-in-memory enablers occupy the quiet middle layer of the neuromorphic stack. They supply the synapses—ReRAM, MRAM, HBM-PIM—that let data stay put while it is processed. When neuromorphic chips scale, the demand for these specialty parts should rise in lockstep, giving investors leverage without the hit-or-miss product cycles of end-system vendors. For those hunting more mature yet still focused neuromorphic computing stocks, this group offers exposure to volume manufacturing and potential licensing royalties.
Weebit Nano (ASX: WBT)
HQ: Israel; Developer of foundry-ready ReRAM for embedded and neuromorphic chips.
Weebit Nano is a small Israeli-Australian chip company that sells a new type of non-volatile memory called ReRAM. In fiscal-year 2024, it booked about US$1 million of license and engineering revenue, yet the more telling milestone was qualifying its first embedded product and taping-out chips with SkyWater and DB HiTek. That momentum underpins the thesis: if ReRAM proves reliable at scale, every microcontroller that now runs on flash could switch over.
Neuromorphic processors need billions of nanoscale “synapses” that can store analog-like weights, update quickly, and sit beside compute cores. Flash is too slow; DRAM leaks power. ReRAM conducts in two stable states and can be programmed to many intermediate levels, so it behaves like a hardware synapse while drawing almost no standby energy. Recent lab work at CEA-Leti and Weebit has already demonstrated few-shot learning directly inside ReRAM arrays.
Investors therefore own an option on two markets: mainstream embedded memory (near-term) and neuromorphic accelerators (long-term). The risks are real too—qualification cycles stretch over years, large foundries are testing their own resistive memories, and Weebit still burns cash. But if even a handful of customers adopt its IP, the resulting stream could scale faster than costs.
Everspin Technologies (NASDAQ: MRAM)
HQ: USA; Sole merchant supplier of MRAM for edge AI and CXL memory.
Everspin Technologies makes magnetoresistive RAM (MRAM), a memory that holds data without power yet writes almost as fast as SRAM. The company produced roughly $50 million in revenue in 2024, sells both discrete chips and IP, ships through GlobalFoundries, and recently secured a $10.5 million U.S. award to embed its MRAM in Purdue’s in-memory-compute project.
Neuromorphic processors need billions of energy-thrifty “weights” right beside the logic core. MRAM fits. Each magnetic tunnel junction flips in nanoseconds, survives trillions of writes, and remembers for decades. Researchers have already built multi-level MRAM synapses and spin-tronic arrays that perform content-addressable searches at one-tenth the power of DRAM, showing MRAM can serve as both storage and compute fabric in a single layer.
Near-term, MRAM can replace battery-hungry flash in industrial IoT and automotive data loggers. Long-term, the same physics could anchor MRAM-based neural accelerators. The firm ended 2024 with $42 million in cash and no debt, giving it time to ride qualification cycles. Risks remain—lumpy defense programs, low market share, and deep-pocketed rivals from Samsung to ferroelectric startups—but if MRAM becomes the default non-volatile latch inside edge-AI chips, Everspin’s royalty stream could scale considerably.
GSI Technology (NASDAQ: GSIT)
HQ: USA; Compute-in-memory firm focused on associative AI processors.
GSI Technology is a 30-year-old Silicon Valley memory company that once lived off high-speed SRAMs for telecom and space. In fiscal-year 2025, it booked about $20 million of revenue and ended with $13 million in cash and no debt, buying it time for a pivot.
That pivot is Gemini, an “associative processing unit” that sprinkles simple logic beside every SRAM cell and lets the whole array act like a content-addressable brain. Data stay where they are; terabytes of vectors can be searched in microseconds without shuttling bits across a bus. Gemini boards, rated at 1.2 peta-operations per second, are already shipping to defense programs and search-engine labs.
Neuromorphic computing is a key avenue for growth. Brains solve problems by making billions of parallel, in-memory comparisons; Gemini recreates that trick in standard CMOS. Each cell can hold multi-bit “weights,” flip in a single cycle, and leak almost no power—qualities that map neatly onto spiking-neural accelerators, event-based cameras, and other edge-AI tasks.
If compute-in-memory becomes essential for vector search and embedded AI, GSI could own a defensible niche and license Gemini IP at software-like margins. If GPUs keep winning or cash runs out first, equity value could shrink quickly.
SK Hynix (KRX: 000660)
HQ: South Korea; DRAM giant advancing PIM for AI and neuromorphic workloads.
SK Hynix is the world’s No. 2 memory maker and the clear leader in high-bandwidth DRAM used in today’s AI servers. In 2024, it generated ₩66 trillion (~US$48 billion) in sales and swung back to operating profit as HBM3E shipments ramped.
That scale funds a second, quieter bet: turning memory arrays into miniature processors. The company’s GDDR6-“AiM” chip embeds simple MAC engines beside every DRAM bank, letting the device perform vector-multiply tasks in situ and cut data movement by up to 90 percent. Engineers have since shown compute-in-memory test dies that hold analog weights, accumulate multi-bit results, and even fire spiking-neuron primitives—exactly the building blocks neuromorphic hardware needs. At CES 2025 SK Hynix spotlighted these AI-centric memories as its next growth pillar.
Near-term, HBM’s supply bottleneck and Nvidia’s insatiable demand give SK Hynix pricing power and cash flow to pay down debt and keep capex high. Longer-term, if neuromorphic accelerators move from labs to edge devices, SK Hynix is one of only three companies able to fabricate the specialized in-memory silicon at scale. Risks are memory cyclicality, massive capital intensity, and Samsung’s push to retake HBM share. But if compute-in-memory goes from demo to design-win, SK Hynix could own the foundry, the IP, and the margin.
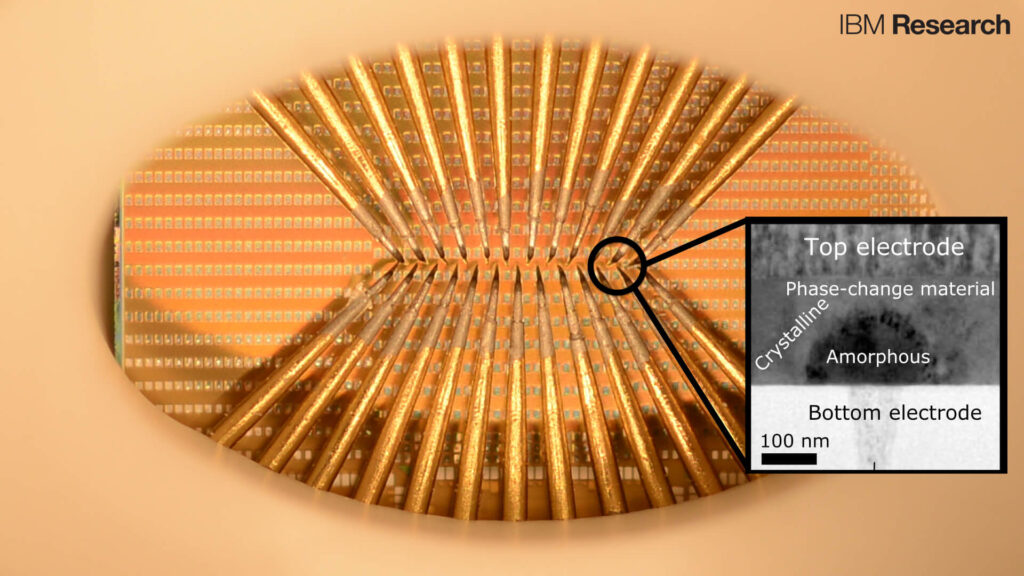
Tech Giants with Neuromorphic R&D
Big-cap tech names with neuromorphic R&D give investors a safer—but more diluted—way to ride the theme. Intel, IBM, and Qualcomm bankroll skunk-works chips like Loihi or NorthPole, so any breakthrough is a free option layered on proven businesses in CPUs, cloud, or wireless. These neuromorphic computing stocks trade on earnings, not press releases, yet they still pursue cutting-edge research. The payoff is slower, but downside is cushioned by diversified revenue streams and deep manufacturing moats.
Intel Corp. (NASDAQ: INTC)
HQ: USA; Developing Loihi, a neuromorphic chip with open-source support.
Intel is best known for x86 CPUs, yet its neuromorphic program may be the most audacious line of research inside the company. The Loihi 2 chip, released through Intel Labs, delivers up to a ten-fold performance boost over the first generation and ships with the open-source Lava software stack so academics and start-ups can tinker without lock-in. In April 2024, Intel wired 1,152 Loihi 2 dies into a six-rack computer dubbed Hala Point that simulates 1.15 billion spiking neurons while drawing only a few kilowatts—roughly the power budget of a household oven.
The bull thesis is leverage. Intel spends billions on process technology and can rapidly cost-reduce once a research device shows commercial promise. If sparse LLM inference or event-based vision moves from labs to edge servers or “AI PCs,” Intel owns the stack: design tools, silicon, and future foundry capacity. Winning even a niche share of accelerators could reinforce the turnaround narrative and diversify revenue beyond PCs. Importantly, the node uses Intel 3 process, showcasing manufacturing leadership.
Yet commercialization remains speculative. Hala Point is still a research box and there is no public product roadmap with attach rates or margins. Intel is juggling multiple bets—Gaudi GPUs, Core Ultra NPUs, silicon photonics—while free cash flow is tight. Competitors may lock customers into rival tool-chains before Lava matures. For now Loihi should be viewed as a free, long-dated option inside Intel’s vast portfolio; exciting, but easy to overhype.
IBM Corp. (NYSE: IBM)
HQ: USA; Researching NorthPole, a low-power vision chip with brain-like design.
IBM pioneered neuromorphic hardware a decade ago with TrueNorth and is now iterating with NorthPole, a 22-billion-transistor prototype that fuses memory and compute on the same die to deliver 13 TB/s of on-chip bandwidth. The chip achieves GPU-class vision inference in single-digit watts and anchors a broader AIU family under development at IBM Research.
The strategy meshes neatly with IBM’s hybrid-cloud pitch. A low-power accelerator that can sit beside Power servers or in OpenShift clusters cuts energy bills and addresses data-sovereignty rules, giving Big Blue a differentiated hardware-plus-software bundle that matches its consulting DNA. Much of the R&D was funded by U.S. defense agencies, meaning IBM could monetize via licensing without heavy fab outlays. Moreover, IBM’s latest annual research letter also flagged a plan to tape-out a scaled NorthPole test chip on a 5 nm node during 2026. Investors who think energy will become the gating factor for AI at scale may welcome that optionality.
Still, the commercial path is fuzzy. NorthPole is a lab device and IBM has no high-volume manufacturing partner; it would rely on foundries it cannot directly influence. Management must choose whether to scale internally, spin the effort out, or license the IP. IBM also has a history of moonshots that stall before material revenue. Without a dated roadmap, reference customers, and third-party benchmarks, neuromorphic success remains a strategic call option rather than a near-term driver—worth watching, not chasing.
Qualcomm Inc. (NASDAQ: QCOM)
HQ: USA; Integrating neuromorphic techniques into mobile and inference NPUs.
Qualcomm attacks neuromorphic computing from the other side of the market: mobile first. The original Zeroth project introduced spiking-network ideas to smartphones; those lessons now underpin the Hexagon NPU in every Snapdragon and the discrete Cloud AI 100 accelerator family. This spring, Dell even announced a workstation laptop with a plug-in AI 100 inference card, giving developers brain-like efficiency in a familiar PCIe form factor.
The bull thesis is volume. Qualcomm already ships hundreds of millions of chipsets a year, so any incremental efficiency flows straight to battery life—an outcome OEMs will pay for. If neuromorphic blocks unlock another two-to-three-fold gain in always-on vision or speech recognition, Snapdragon could widen its lead in the power envelopes most prized by handset makers. In the data center, Cloud AI 100 targets cost-optimized inference where watts per token matter more than peak FLOPS, positioning Qualcomm as a low-carbon supplier in a crowded field.
The bear view centers on focus. 5G modem royalties still bankroll most R&D, and management must spread capital across automotive, XR, and RISC-V ventures as well as neuromorphic hardware. NPUs also lack an entrenched software moat; if Qualcomm’s tool-chain lags, developers will default to CUDA or Triton. Larger rivals can bundle NPUs at near-zero margin to gain share. Qualcomm’s neuromorphic push therefore looks like a calculated option—potentially valuable, but not yet the crown jewel. Watch for real-world benchmarks in Dell’s machines late this year.
Private Neuromorphic Companies to Watch
Neuromorphic computing is an incredibly young field, and many of the most promising startups are still private. In addition to BrainChip (which is already public), we cover five other innovative startups—SynSense, GrAI Matter Lab, Prophesee, Innatera, and MemComputing—on this page.