What if machines could learn from experience, adapt to the unexpected, and operate on a fraction of the energy they consume today? Would that be true machine intelligence? No, we’re not talking about today’s AI existing algorithms. We’re talking about new computer chips built from-the-ground-up to physically mimic the human brain. In this article, we explore 6 promising neuromorphic computing startups that could take us to the next frontier of artificial intelligence.
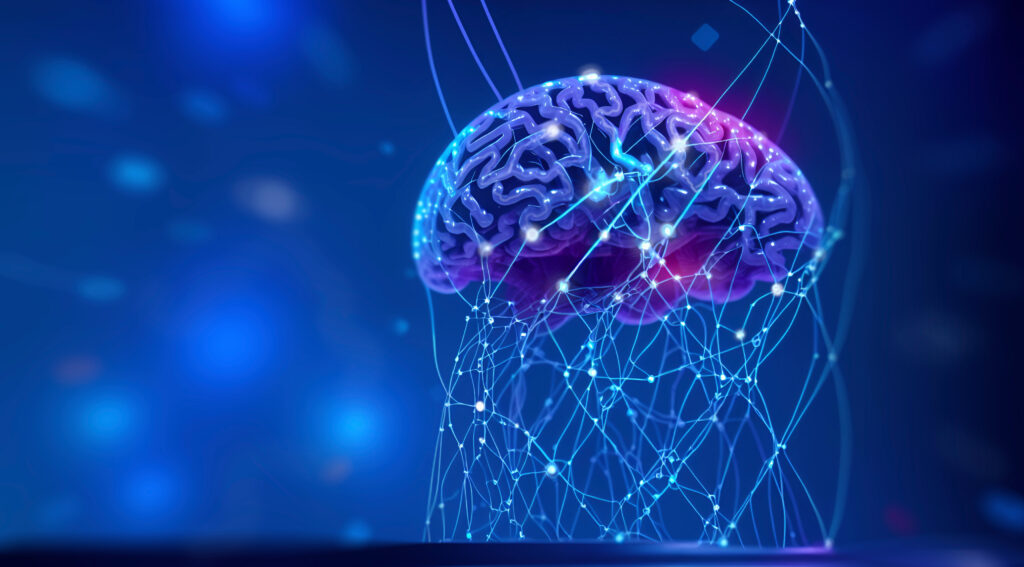
What makes neuromorphic computing so special?
Regular computers process information like following a recipe: they take instructions, one step at a time, until they get an answer. It’s like following a long list of steps to solve a math problem.
Neuromorphic computing is different. It’s inspired by the brain, which is like a giant web of connections between tiny processing units called neurons. These connections can get stronger or weaker based on the information that flows through them.
In simple terms, neuromorphic computers are built to mimic this. They have artificial “neurons” connected in a web, and these connections can change based on the information they receive. This allows them to learn and adapt over time, without needing a long list of instructions.
This is different from deep learning AI, which is still designed to run on traditional hardware. Neuromorphic computing aims to fundamentally change how the hardware works. This could in turn lead to major breakthroughs in AI, especially in areas where power efficiency and on-the-fly adaptation are critical.
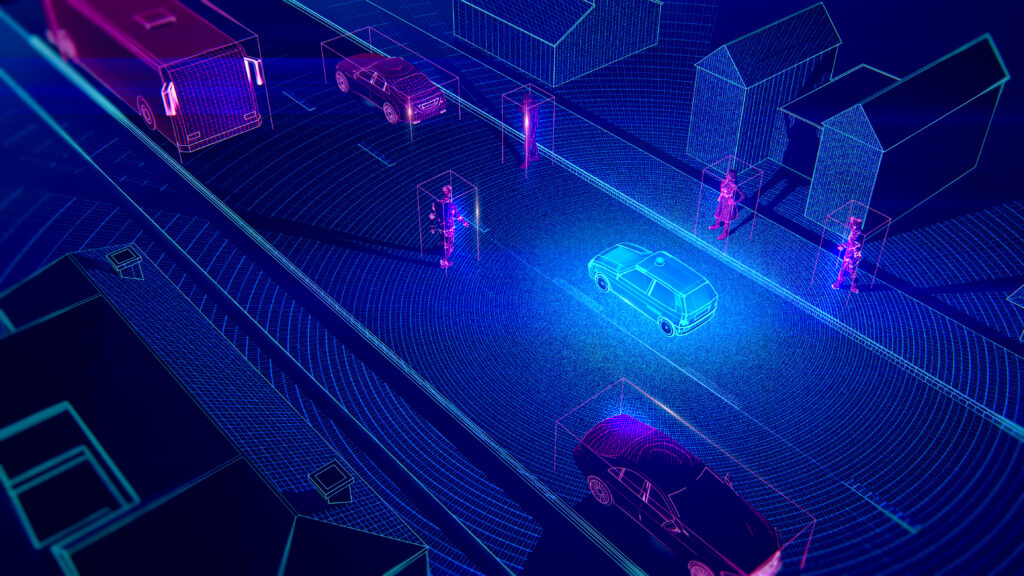
Let’s look at some of the neuromorphic computing startups leading the charge in this exciting space.
BrainChip
BrainChip, headquartered in Aliso Viejo, California, is a leading force in neuromorphic computing. Founded in 2004, Brainchip is a leader in bringing brain-like efficiency to edge AI, and they are unique for their established commercial partnerships. Their core product is the Akida neuromorphic processor, which is designed for low-power yet powerful edge AI.
Here’s what makes BrainChip’s Akida neuromorphic processor unique:
- Spiking Neural Networks (SNNs): Unlike most AI systems that rely on artificial neural networks, Akida uses SNNs. These networks more closely mimic the brain’s communication patterns. Neurons fire brief “spikes” of activity only when needed, leading to great energy efficiency.
- Event-Based Processing: Akida chips are designed to react to changes in data rather than constantly processing every single data point. Imagine a security camera that stays dormant until motion triggers it – this is akin to how Akida saves power.
- On-Chip Learning: Part of Akida’s power lies in its ability to learn and adapt without needing to send data back to the cloud. This is critical for edge devices that require data privacy.
BrainChip’s low-power edge AI solutions have a broad range of applications. The most obvious are always-on smart home, factory, or city sensors. Their chips could go into vision, sound, or vibration detectors that need to last years on a single charge. Robots and autonomous vehicles also need powerful AI that consumes minimal power. Akida chips also have use cases in cybersecurity for detecting subtle anomalies in network patterns that traditional systems might overlook.
SynSense
SynSense (formerly aiCTX) is a Swiss-based company at the forefront of neuromorphic computing. Founded in 2017, they are at the forefront of ultra-low-power vision and sensor fusion solutions, with a singular focus on mimicking how we see the world. SynSense draws on decades of academic research on biologically-inspired neuromorphic processing. They develop both neuromorphic vision sensors and dedicated low-power AI processing chips.
SynSense’s approach to neuromorphic computing centers on how we see the world:
- Event-Based Vision: Like BrainChip, SynSense leverages the idea of event-based processing. Their Dynamic Vision Sensors (DVS) don’t capture traditional image frames like a classic camera. Instead, they respond to changes in brightness. This means pixels only activate when they see movement, which saves massive amounts of power and data.
- Sensor + Processor Fusion: SynSense doesn’t just build sensors. They also have neuromorphic processors designed to handle the kind of data these sensors generate. This tight integration leads to very low latency and power consumption.
- Real-Time Understanding: Their technology enables devices to understand the visual scene in real-time. This means tracking objects, detecting movement, and responding instantly, all with minimal power.
SynSense’s technology has valuable use cases in various fields where machine vision is vital. In factories, their sensors and processors could enhance machine vision systems. They can dramatically improve core tasks precision counting, flagging defects, or tracking parts. For robots and drones, SynSense’s solutions offer a way to achieve real-time, efficient vision. This is crucial for navigating complex environments and avoiding obstacles without draining batteries.
SynSense vs. Brainchip: While both BrainChip and SynSense leverage the power of event-based processing, SynSense’s specialization in computer vision sets them apart. Their Dynamic Vision Sensors and processors focus specifically on enabling devices to ‘see’ the world in an energy-efficient way, potentially narrowing their focus compared to BrainChip’s more general AI applications.
Prophesee
Paris-based Prophesee is another leader in event-based neuromorphic computing for machine vision. Founded in 2014, they are pioneers in developing event-based vision sensors. They have partnerships with major players, including Sony, Bosch, Renault, and Xiaomi.
Prophesee’s core innovation is their Metavision event-based vision sensors. Here’s what makes them unique:
- Pixel Independence: Each pixel in a Metavision sensor operates independently. Instead of capturing frames at a fixed rate, pixels only activate when they detect a change in brightness within their field of view.
- High Temporal Resolution: This independent operation gives Metavision sensors an incredibly high temporal resolution (measured in microseconds). That means they can capture extremely fine-grained motion that traditional cameras would miss.
- Low Data Output & Power Consumption: Only pixels that “see” a change generate data. Thus, their sensors have much lower data output and power consumption compared to conventional cameras.
- High Dynamic Range: Their sensors can function in extremely challenging lighting conditions—from near-darkness to very bright light—without the saturation issues faced by standard cameras.
Prophesee’s event-based vision offers a fresh approach to surveillance. Their sensors can catch details that standard cameras miss, especially when things happen quickly. They work amazingly well in low light, so darkness won’t limit what they see. Since they only react to real changes, bothersome false alarms from shadows or small movements could be reduced. Finally, they might open the door to surveillance that better respects privacy, as data wouldn’t need to be uploaded to the cloud.
Innatera
Founded in 2018, Innatera is a Dutch startup making significant strides in neuromorphic computing. Their core technology centers around ultra-low-power analog neuromorphic processors. Innatera specifically focuses on advanced cognitive applications enabled by spiking neural networks (SNNs).
Innatera leverages both sophisticated spiking neural networks and a unique analog computing approach. Here’s how:
- Spiking Neural Networks (SNNs): Like some other startups, Innatera uses biologically-inspired SNNs. These networks use precisely-timed events (spikes), like how neurons in our brains communicate. This event-based approach translates to extreme energy efficiency.
- Analog Computing: Instead of digital processors, Innatera uses analog circuits. Designed specifically for SNNs, these analog circuits are another major source of power savings. They’re especially useful for the complex calculations needed within neural networks.
- Advanced Cognition: Traditional AI systems often excel at tasks like classifying images (is this a cat or a dog?). Innatera’s goal is to go far beyond that. Think of making predictions in dynamic environments where standard algorithmic approaches might struggle.
Innatera’s technology has use cases in several high-value fields, such as finance. Vast amounts of data, including news, sentiment, and trading behavior, interact in subtle ways to influence market trends. Traditional algorithms often struggle to spot these nuanced relationships. Innatera’s focus on pattern recognition within complex, noisy data could be game-changing here. Markets are also volatile. Innatera’s chips, designed for real-time adaptation, could enable systems to react with unparalleled speed. This gives them an advantage when every millisecond matters.
Innatera vs. GrAI Matter Labs: Both Innatera and GrAI Matter Labs utilize spiking neural networks for efficiency. However, Innatera’s emphasis on pushing the boundaries of complex cognition sets them apart. Additionally, Innatera uses a fully analog approach, while GML incorporates elements of traditional deep learning, potentially impacting both their capabilities and limitations.
MemComputing
Founded in 2016, MemComputing is a San Diego-based startup with a truly unique approach to neuromorphic computing. Unlike the companies we’ve discussed so far, MemComputing leverages physics-based computing principles. Their technology centers around “memcomputing” processors designed for complex optimization problems.
MemComputing breaks away from traditional computing architectures in several fundamental ways:
- Physics-Based Computing: Their processors don’t rely on transistors like standard computers. Instead, they leverage the natural behavior of physical systems that evolve to find the lowest energy state. This can be used to represent the optimal solution to a problem.
- Solving Optimization Problems: MemComputing focuses on combinatorial optimization problems. This is a specific, but valuable category of problems. It involves finding the best arrangement or choices from a vast number of possibilities.
- Non-von Neumann Architecture: Most computers today follow the von Neumann architecture. This model has memory (where data is stored) and processing (where calculations are done) as distinct units. Traditional computers separate memory and processing. This can lead to bottlenecks, as data needs to constantly shuttle back and forth between memory and the processor. MemComputing processors blur that line. They perform computations directly where data is stored, reducing the constant back-and-forth.
MemComputing’s technology finds its niche in tackling complex optimization problems. This includes things like finding the most efficient route for a fleet of delivery vehicles, with dozens of stops and ever-changing traffic conditions. Or allocating resources in a connected smart city. Scientific fields like drug discovery and materials science would especially benefit.
—
This field is still young, but it holds the key to a future where computers are more intuitive, yet use less power. As the world continues to chase high-energy-usage computing like AI and blockchain, the energy savings offered by neuromorphic processors will become increasingly important.
That said, neuromorphic computing isn’t just about efficiency. It’s about mimicking the human brain’s incredible ability to learn and adapt. As this technology matures, it could pave the way for a new generation of AI. And if it reaches its full potential, it could even become a pivotal unlock along the path to artificial general intelligence (AGI). These neuromorphic computing startups are working to make that happen.